Efraim Racker

Enabling a Predictive, Preventive and Personalized Medicine with Striking Opportunities and Challenges for Society
Transcript
[ Silence ] >> Totally changed the way we think about problems.
So where did the guy did this come from?
Well, Lee was born in Missoula, Montana.
He went to Caltech as an undergraduate, got his MD degree at Johns Hopkins University and then went back to get a PhD in biochemistry at Caltech University where he was on the faculty on the biology department for 22 years, from 1970 to 1992.
And during that time, he spent a good fraction of his efforts working on technology development and that technology development actually resulted in formation of a number of companies including Applied Biosystems, one of our favorites.
And when he was at Caltech, he had the opportunity to form a new company, a new department that was actually able to realize all the visions of new ways of approaching problems at the University of Washington.
That was the Department of Molecular Biotechnology.
He was there from 1992 to 2000.
In 2000, they changed a little bit the University of Washington and he formed yet another group that we're going to hear a lot about in the seminar today which is the Institute for Systems Biology.
Now, systems biology is sort of a different way of thinking about the life sciences.
It's very integrated.
It's a little bit hard to pin down.
I think the best way to define systems biology that I come across is to say Lee Hood and look at the website for his institute.
And so today we're going to hear Systems Biology, Transforming Biology and Medicine.
[ Pause ]
- >> Lee Hood
There are two seats up here, if anyone would like to.
What I'd like to talk about today is exactly that topic namely, exactly my view of systems biology and then we'll show two general complications of systems biology, one to a fundamental problem in biology and one to a fundamental problem in medicine.
So let me start by defining systems biology.
The system that you would like to say will be defined by the biologic phenotypic traits that you're interested in.
And basically what you can do is draw a box around a set of genes, a set of proteins that encode, enable a particular kind of trait.
What you want to do is one, define all of the elements that are components of that particular system, generally proteins.
And number two, you want to determine their interrelationships, their associations, their-- the influences they have upon one another.
And we can display systems in graphical terms, these networks as I've shown you here where the nodes or the proteins, for example, and the lines or the nature of the associations and so forth.
What was transformational about the human genome project is it enabled us to view systems in context.
So if we perturb the elements of the system, we not only could ascertain how the other elements in that system responded to that perturbation but we could look at how that system communicated with and talked to many other systems in whatever the biological community or study was.
It could be the cell, it could be a whole organism.
But what is very important to realize about biological systems is they are enormously dynamic and that represents one of the striking challenges in systems biology.
How do you represent these, the dynamic nature of these networks and their behavior?
The idea then is if we understand elements and interactions and if we understand context in time, we'll be able to explain the system's properties, the emergent properties of the system and you know, this all sounds like a very straightforward kind of thing.
When I go around and give lectures like this, all that people say, but wait a minute, were the physiologists of hundred plus years ago interested in the systems and neurobiologists and the immunologists, and the answer is of course, they were interested in systems.
But what differentiates these systems biology from those systems approaches I think are three really fundamental things.
One, because of the genome project we can do global analyses where global means we can look at all genes in principle, all polymorphisms, the entire transcription of all the message levels, we can look in principle again in proteins.
We can't do it ideally but we will in time be able to do that as well.
So we can carry out these global analyses but systems biology is something more.
Embedded in it is the idea that you can never truly understand systems unless you integrate different levels of information, of biological information, the DNA level, the RNA level, the protein level, the network level and so forth.
And so this idea of integrating information is the second idea that's fundamental to systems biology.
And of course the third idea is that inherent in the first idea, first two ideas, is the idea that you need to be able to make millions and we'll see later on humans, probably billions of measurements on systems.
And so, one of the fundamental problems in systems biology is the problem of how you reduce the information dimensionality from billions to coherent hypotheses about how particular biological system work and that again, is an enormously fascinating challenge of systems biology.
Now, second aspect of systems biology is the realization, number 1, it's a very immature science and number 2, that-- as a consequence, those groups that I think are really doing effective systems biology are using the dictates and the needs of systems biology to grow the development of these technologies [inaudible] technologies.
And these in turn with their capacity to generate large global datasets mandate the need to drive new, the development of new computational and mathematical tools, and these all work empirically together to-- in a very, very powerful and synergistic fashion.
What's necessary for doing that is creating something, I think Cornell has uniquely in many ways that is a true cross-disciplinary culture.
And by cross-disciplinary, I mean a number of things.
I mean one, scientists who have learned to speak the language of their other colleagues.
I mean two, non-biologists who will learn what they're doing in biology in a very deep sense so they won't merely end up being technicians.
And three, I mean, that in systems biology, there is a real mandate to focus on a few problems because these are really difficult problems.
And of course if you succeed then you can generate biological information.
Now, when I started thinking about technology back in 1970 when I started at Caltech, actually.
My vision of what technology was all about was deciphering biological information.
And I think what has happened particularly with the the human genome project is-- it has come into a much clearer focus, this idea that biology is most fundamentally an information science.
And if you think about it that way, that is the most efficient way to teach and to learn what biology is all about.
So let me give you just a quick primer on, you know, how I view biology as an informational science.
I would argue first that there are two fundamental types of biological information.
There's the digital information that's encoded in the core of all life, our DNA, our genome.
And of course, there is the environmental cues that come in from the outside and tap on that DNA and modify the output of the information that comes from that DNA.
And indeed, what biology is really all about is, and particularly what systems biology is all about, is understanding the inner digitation, the integration of these two types of information across three different dimensions of life, evolution, development and physiological responses.
I think what's really interesting about the digital core of life is it uniquely distinguishes biology from all other scientific disciplines because there is no other scientific discipline for which the core set of information is digital and counts, in principle, ultimately notable.
And I think that has really interesting implications.
Now, if we think about the digital information, the genome, I would argue that there are really two fundamental types of networks that are encoded in the genome.
The first, of course, are something you all know, these are the protein networks, proteins interacting with other proteins that's interacting with small molecules.
These create signal transduction network.
They create batteries of genes that carry out development or physiology, batteries of gene that carry out metabolism and so forth.
And the second type of network is one that fewer people really understand in detail and it arises from the idea that there are types of proteins interacting with control elements, cis-control elements that regulate the expression of genes.
And in fact, if you have transcription factors regulating the expression of other transcription factors, what you then operationally end up generating are layers of transcription factors that are regulated in a positive or a negative sense by very complicated feedback control mechanisms, but, and these constitute gene regulatory networks.
They are the grand integrating and modulating networks of biological information.
You input signal from the signal transduction pathways.
You modulate it.
You change it.
You output it to the batteries of genes that carry out either development or differentiation.
And here's the most complicated gene regulatory network we know about today is this, something we worked on with Eric Davidson for the past 25 years.
It is about 50 transcription factor gene element network that encodes endomesodermal development in the sea urchin.
And I'm not going to talk about the biology here except to make two points.
This network tells us enormous detail and with enormous precision the events that transpire in the development of effective mesoderm during the first 72 hours of development of the sea urchin larva.
So much so that we-- that Eric and his coworkers have actually been able to reengineer this network so it created completely new emergent properties.
For example, he's reengineered the network to create sea urchin that predictably had two guts rather than one gut and reengineered it to predictably convert all pigment cells into skeleton cells.
And the reason I tell you this is that in the future medicine is going to be about the reengineering of disease perturbed networks with multiple drugs so that those networks will behave in a more normal fashion and this is the context for which we'll have a later discussion about this new approach to drug discovery.
The final point I would make is that information is hierarchical.
We go from DNA to RNA to proteins, to interactions, to networks and cells and on down.
And the really key point again is this point of integration namely, at each of those informational levels, environmental information impinges upon and changes the fundamental digital output and that means if we're to truly understand biological systems we have to be able to capture as many different levels of biological information and integrate them together in ways we'll talk about in a few moments.
So, with that as a context let me then tell you a little bit about what we're doing and what we've done over the pass five years at the Institute for Systems Biology for handling some of these problems of the integration and management and ultimately modeling.
Our view of how we model biological information is that it must be empirically driven by data.
So, one of the prerequisites was to create really effective database which we found that now can handle about 15 different types of global datasets.
And in fact, we have the ability to integrate individual elements from those global datasets and integrate the literature and bring in information on those.
But most important of all, we have the ability to use this basic raw data with programs that can integrate this information together in the form of network hypothesis about how the biology works.
The more data that's integrated together the more sharply articulated are these networks and the more closely they resemble the hypotheses that have to explain the biology and so forth.
This is a program we developed at the institute.
All the institute software is open source.
It's called Cytoscape and it is kind of one of the basic tools now for the integration and hypothesis formulation in systems biology.
For small systems that we have lots of information, we can write logic backgrounds and you can write nice differential equations that explain the behavior.
One of the questions that I have raised is in the more complicated systems, "Can we use differential equations to actually describe their behavior?" I mean, one aspect is, how do you define all the parameters and the multiplicity of differential equations you need?
But a second I think much more fundamental issue is this question of how can you reflect in differential equations the integration of these very different types of data?
So there may be a need to think about new approaches to mathematical representation of biological models.
We have put together pipelines that allow us to carry out, you know, very specific weight proteomics and DNA array kinds of analyses.
We have put together many different kinds of analytic programs that do various things, biochemistry allows us to practically display which you'll see later gene regulatory networks.
And populace is a program for-- we actually just submitted two papers to PNA.
It has given as a means of statistically integrating multiple datasets.
In fact, in one of the examples we used, we integrated 18 different datasets to form, again, graphical models about the nature of the biology that we were interested in.
And all of these programs go through the database and through the analytic program and ultimately feed into the site [inaudible] related kinds of things.
But I thought what might be interesting today is to talk about one model of organism that we've been interested in since the inception of the institution, show not so much about the biology 'cause it's an incredibly fascinating biology that will take a whole lecture to get to the biology, but to show how it's mandated and driven the need for new computational tools.
So the creature is a halobacteria, an archaea bacteria, that lives in five molar salt, that's ten times the concentration you live in.
And it has some really interesting properties.
It is an enormously radiation-resistant.
It lives in very high salt concentrations.
It has incredibly stable enzymes.
So, we're interested in reengineering this organism to be able to do bioremediation, and actually, is a very efficient transducer of something like in energy, so bio-energy.
And to do it, we obviously have to understand its protein and gene regulatory networks.
It's a small genome, 2,700 genes or so forth.
It has three sets of chromosomes and so forth.
And here's what's known about it when we started our studies just before the beginning of 2000, there were two little systems that have been studied to a certain extent in the halobacterium, and together with several colleagues and including most recently Nitin Baliga, we have, over the past five years, studied this organism, sequenced its genome.
We've sequenced the genome of a related organism and we've carried out full series of studies, some of which, I'll highlight.
But the basic philosophy has been, again, if you use this organism to drive the development of new technologies, particularly in this case, new computational technologies.
So, we hunt what could be a good way to start would be to interrogate in some detail the 13 different basal transcription factors that exist in two discrete classes in this organism.
And there's some hint that they may be employed in a combinatorial fashion as generating even at the basal transcription factor in the norm of the lateral potential diversity and how transcription factors can interact with a system called Elements.
What we have done then on the one hand is to look at a whole series of environmental permutations that relate to things that we're interested in.
And we've done more than 400 dynamic transitional analyses that now actually encompass close to 2 million data points.
This is an over-slide.
So the question is, how do you deal with 2 million data points worth of transcriptional data?
And what we've also done is a lot of protein-protein interaction data.
We've looked at a lot of proteomic data using techniques that allow you to quantitate lots of changes in different dynamic permutations.
And we carried out analyses that permit us to delineate protein DNA interactions.
And, again, that is an enormous amount of data and how do you go about analyzing that in such an organism.
Well, to do it, we've developed three new programs which I'll talk about too here.
One is the program called cMonkey and its objective is to find co-regulated groups of genes.
And I mean co-regulated, not fully expressed, co-regulated means they share the same transcriptional factor strategy for regulation.
And what this program enables us to do is to take that enormous amount of transcript data and to cluster it so that you can see across which dynamic transitions, certain subsets of genes, are co-regulated.
And the behavior of different genes changes across different conditions in very, very interesting ways.
And, in addition, this program has the ability to find the cis-regulatory genes and other kinds of things.
What this program can do then is feed that information to a program called the Interpolator, and this is a statistical-based program that can take enormous amounts of different kinds of data and it ascertains likely probabilistic relationships between key gene regulatory factors and balance the chains that they actually regulate.
And of course, what we can do then are experimental permutations to test the ideas that eventually come from these regulators.
And here's the cMonkey program.
It allows us to do a clustering of genes that operate together.
It allows us [inaudible] in all of these things to identify motifs that are commonly shared by clustered sets of genes and so forth.
And it allows us to look at relational networks that relate to the special gene organization of archaea bacteria namely operons, have inferential implications about how those genes shared in the operons are actually co-regulated and so forth.
What this is is a first shot at the protein gene regulatory networks that come from the data that was processed by cMonkey and was analyzed by the Interpolator.
And the Interpolator identifies two kinds of key relationships, namely, the relationships between key transcription factors that regulate patterns of genes, and the relationships between environmental factors that also can regulate the networks of genes and so forth.
And we'll focus down on this gene-regulatory network in just a moment.
But to make one other point about analyzing very large amounts of data, if we put together all of the things that we have, another major question comes up and that is, you like to be able to take particular subsets of genes and to survey them across all the different dimensions of the informational hierarchy you have.
And for that, Nitin Baliga, Paul Shannon and Michael Johnson actually developed a program called the Gaggle bonds which is interconnected to all of the information that's present in this system, the enormous amounts of information.
And what you have the ability to do within Gaggle bonds is pick out some subset of genes that you're interested in and it will communicate with all of the other data sets and it will ask them to display respectively those subsets of genes in the context of whatever informational analyses they've done.
And this is absolutely critical for going from the big and daring walls which are these walls of gene and regulatory networks and to looking specifically at the biology of individual tasks.
So, getting back to mapping out all of these global transcription factors, it turns out that we could map all of one site and not most of another [inaudible] just for technical reasons.
But we were able to show that about two-thirds of these general transcription factors have mapped to roughly half the genes and so forth, and we were able to uniquely assign their mapping points and so forth.
And it began to give us then the input to create the gene-regulatory networks that my-- our heart of all of this process of big integration.
I will say in prokaryote-- in turkey organisms, the gene regulatory networks are enormously shallow in their dimensions.
So, there's a lot of this integration than a more complicated kinds of organisms.
And here is regulation of transcription factors and other factors such as oxygen and so forth that regulate the battery of genes that may provide us all new protein.
So, we can pull out this set and we can, in an instant, see how these things are coordinately regulated and what key regulators are and so forth.
And this, of course, is really important if you're going to think about starting to redesign the networks.
And there's a most recent work done in a NiPS lab, and basically, what he's done is a series of permutations with most common kinds of [inaudible].
And what he was able to demonstrate here beautifully is that about half the mechanisms for having [inaudible], and this is obviously really important for bioremediation, are classical mechanisms that you've seen before in other organisms, and by our account are really unique new kinds of organisms.
And what I want to point out is with the tools we've developed here, we not only can do the overall global analyses of these things but you can hone in very specifically on very subtle details of biology.
So, systems biology is holistic in the sense that you can look in the context of a whole organism, but it's also reductionistic in the sense that you can focus on the very specific graphical details, in particular, biological systems.
Now, the point I like you to take away that I think is most important and fundamental is the idea the data space is infinite.
What that means is if you're interested in a particular biological system, you have to formulate your hypothesis in a very clear way so you will illuminate that part of data space which is relevant to your system.
In this regard, just let me say, lots of biologists say, "Well, if it's me, where do I begin to deal with all of these global data sets out there?" What I would say is, many of those data sets are not going to be relevant to your biology at all, and in fact, that they could be very misleading because they're taken in the wrong connection of data space.
And the implication is that systems biology has enormous amount to offer to people doing small sciences all the way up to science later.
And in fact, it is really imperative that universities such as Cornell have the capacity to get those people doing small science access to the ability to probe the data spaces that are going to be relevant to their own smaller systems and so forth.
And of course, you know, one of the questions I would just reiterate again is this idea that you've seen for millions and millions of measurements we've been talking about here, again, the problem that we have to come to through software programs, the cis-reduction and information dimensionality, the creation of hypothesis that will give us ideas about how to go forward and understanding the system or gateway.
So, that's the end of introduction, let me then talk about systems approach to disease.
And at the first instance, the idea is really the simple one namely there are one or more networks in the disease organisms that are either-- and genetically or environmentally perturbed.
And as a consequence, they alter their patterns of gene expression.
Now, this has really, really interesting implications with regard to disease.
If that really is true, if disease does arise from one or more perturbed networks, then the gene whose expression patterns of control, or at least some of them that are controlled by these networks, as I've said, are altered.
And what is very interesting is of why are there each in higher organisms but-- and also in human, 10 to 15 percent of the genes that are controlled by any network are actually secreted into the blood.
And the really important point to understand is that there they constitute molecular fingerprints that reflect the state of the organ from which they are secreted.
And the really important new idea for diagnostics then is every single organ in a human must have these reflecting molecular fingerprints, and if you could read them, you would have a completely new approach to assessing health and disease in the human organs, and that's a lot of what I'm going to talk about.
The final point is that because these proteins whose molecular fingerprints are altered in disease were of quite fundamental network operations in the diseased organ, they can be used as pointers to go back and identify the molecular networks they do might wish to perturb and convert to a more normal activity with drugs.
And, again, we're going to return to that point later.
So, again, the idea is that in studying this kind of disease, you need to make absolutely enormous amounts of numbers of measurements.
And in fact, to set up the kind of thing that I'm going to be talking about in terms of this simple idea of disease diagnostics, we need to make more than a 100 million measurements in prostate cancer or one model system for disease.
And I'm only going to talk about a subset of the studies we've done there because they graphically represent-- they graphically represent two things, one, that in disease, networks are diseased perturb.
And two, during the progression of the disease, those networks change dynamically.
So you not only can make the diagnosis, you can say how far along that the progression line a particular disease work in its column.
So, that let you talk about both dimensions of disease, if you will.
So the idea is we've studied up prostate cancer cell lining called LNCaP, it's androgen-sensitive, and in that sense, it's modeled for early prostate cancer.
And the derivative from this line, it's androgen-insensitive, and in that sense, it's the late-- it's modeled for late prostate cancer.
And what we're going to talk about is how to transcriptome changes from early to late prostate cancer and what implications that has for disease.
Now, the approach that we've taken to doing transcriptome analysis is not DNA arrays, and I have to say, I think DNA arrays have real limitations.
I'm not going to talk about those.
But I am going to tell you about what I think is going to take over in the not too distant future, and that is the ability to use techniques that can do digital accounting of RNA molecules.
And the reason this is important is you can look at multiple changes in a statistically significant way, and you can look at below upon its messenger RNA which are really key to a lot of biology that we have to deal with.
And the technology we use is technology headed Sydney Brenner called Multiple Signature Parallel Sequencing.
And the idea is you can construct from a prostate organ then seed it in a library of a million clones.
You can affix each of those million clones to a different, you know, one of the million feeds and you can amplify them [inaudible] quite bigger than this, more or less.
And then you can rewind those feeds in a closed cell and you can simultaneously sequence a million sequences for 20 residues, and in doing so, you get these signature sequences that from the genome sequence then allowing to identify the gene which encodes the corresponding message that you've analyzed.
So, let me tell you about two kinds of experiments we've done, and we're only going to really talk about one in detail.
So one we can do is take this LNCaP cell line and we can starve it for androgen and we can simulate it with androgen.
So-- and what we can look at then is what changes in that process, and this is the androgen-responsive network in this cell, and that plays a really important role in the biology of the system.
So we've done this to generate the nature of protein network, we've done it to generate major gene regulatory network, and from this, you can say, a lot of interesting biological events.
But what I really like to talk about is another [inaudible] series of studies we've done mainly comparing the transcriptomes of the cell that presents an early prostate cancer in the cell, it represents a late prostate cancer.
And to do these analyses, we've generated 2,000 and-- about 2 million and 3 million MPSS signatures respectively.
And we come to a series of really interesting conclusions.
First, there are about 18,000 genes expressed in these cell types.
We have-- >> Mass?
>> Mass majority are expressed at very low MX levels, but many of them are important biologically.
Out of 18,000, about 2,000 are perturbed in this transition from early to late prostate cancer.
Number two, we can look at the 2,000 that are perturbed and we can map them into protein and gene regulatory networks and represent it in KEGG and Biocarta, and other databases, and we can find that there are about 40 pathways that have been up-regulated or bound-regulated.
And we define up or down-regulated by saying more than half of the protein components that are the-- a messenger components in this case, right in those pathways that changed in a statistically significant fashion up and or down.
And these pathways, many of them are exactly what you think.
As a cancer cell becomes more invasive, then it has an increased ability to break away and become metastatic and so forth.
What is also important is about a fifth of the transcription factors to which you can see 554 have changed, that means the gene regulatory methods are changing, I'll just say, parenthetically, when we looked at these with DNA arrays, we missed more than half of these transcription factors again because they're expressed at these very low levels.
And about 20 percent or so of the messages that changed actually computationally in the code of proteins that are potentially secreted.
So that means they could represent a molecular fingerprint which replaced this whole process.
So, let's then-- so, we've shown you that networks change, we've shown you a few [inaudible] about secreted proteins.
So let's go on and talk about then this idea of blood being a window into health and disease.
And I'm sure as many of you know that there are have been a gazillion meetings about biomarkers that have been presented all over the country.
And I have to say I think almost all the work that's been done on biomarkers is nonsense.
And I think it's nonsense for the following reasons.
It is true if you are interested in a particular disease, you can compare the normal and the prostate cancer disease state and you can find all of sorts of biomarkers that correlate with that change in the state, okay?
The real question is, suppose that we look at 40 other diseases, how many of those biomarkers are we finding uniquely distinguished?
The first disease or between any of those diseases?
And the answer is almost none because most of them are expressed in multiple organs and they'll be perturbed in different ways and different diseases.
So that has been a fatal flaw in creating biomarkers that I've never understood why people didn't correct it.
So, how do we address that fatal flaw?
We address it by taking the transcriptome of the prostate and comparing it to-- through the other transcriptomes where we've got roughly 2 million or more signature sequences for each of the transcriptomes for organs and cell types, and other things that are present in the body, and then we ask how many of the signatures in the prostate are essentially uniquely expressed in the prostate, and we can write this out statistically.
But-- so, we'll say it's predominantly expressed in the prostate.
Again, this is an example of the transcript that is predominantly expressed in the prostate.
And how many do we have map of those?
We have roughly got, well, more than 300 that we've identified out of the 2,000 that has been changed.
And there are 62 of these that are potentially secreted.
So, what we've done now in the two phases, and I'll show you the data from one case, is we'd made antibodies against these [inaudible] prostate cancer secreted markers.
And in one case, what we've done is looked at 10-10 from advanced prostate cancer-- 10-0 from early stage prostate cancer to 10-normals, and with the antibodies to this marker WDR19, we've been able to show that in half of the advanced that we have with the early cases we can detect them [inaudible] not the normal cases.
And we compared it against the classic standard that makes billions of dollars a year as a blood marker for prostate cancer, PSA, and we find it does 7 out of 10 at the advanced, and it does not at the early ones.
But the important point isn't which is the best marker, the important point is, if you put the two markets together, the two of them-- a multi-parameter analysis does much better than either marker alone.
And that I think is really an important point to keep in mind as we're going to about more in just a moment.
And we've actually gone through all of the different organs now, and that's just to say, they're-- these are just the organ-specific markers that are present in various marker cell.
Virtually, every organ is going to give rise to a unique fingerprint, which if you can read those molecular fingerprints, if they change, you'll know that change has occurred in that organ.
So, it's really a very, you know, a simple point.
But let's talk about technology now because how do we bring out these multi-parameter blood diagnostic fingerprints?
And let me just say, I am really convinced that they are really demonstrating informative diagnosis.
Do you have cancer?
Which organ is it in?
Now, where is it?
And, or-- there's an imaging technique that I'll talk about it later.
Is it early stage or late stage?
What's the appropriate therapy?
Should you be on multiple therapies?
Can you look at that person, you know-- all of those things are going to be possible to follow up the fingerprints in the different ways.
We have preliminary data on virtually all of these types of things.
But one big question is, how many markers should we be able to read out of this blood?
My guess is, if we don't know, then for each organ maybe we need 10 to 20 markers to serve as status reports for, you know-- so, if you see a change from the prostate blood fingerprint, our priority to change can represent hypertrophy, it can represent inflammation, or it can represent the one of three or four different major types of prostate cancers.
And I think you need enough markers so we can distinguish all of those common disease, and my guess is that's 10 to 20.
So if you wanted to do 10 to 20 for the top cancers, that would be-- that would get you in the 200 to 400, and if you want to look at lots and lots of other different kinds of diseases in exactly the same way.
So, by the time we get done with all the wish list that we'd like to have, perhaps we need to be able to make a thousand to 2, 000 measurements, and of course the question is, how do we read these blood fingerprints?
Today, we read through our antibodies, and of course, my argument is antibodies at least as we make them today are not going to be on their way to be able to detect on the scale we're talking about.
So, the scale we're talking about is two diagnoses per year for every person at the United States and the European community, in Asia, and things like that, for routine blood diagnostics.
So, you're talking-- you're talking about billions of measurements, and currently, you're not going to do it with the simple protein-capturing methods that we know about today.
Proteomics, what proteomics really are, technically, there are enormous difficulties, it isn't nearly as sensitive as the antibodies are, but I think it's going to be a very powerful technique in discovering.
It isn't going to be a large-scale technique, and I think the only way to do it is to use microfluidic and nanotechnology techniques.
And that's really what we'll talk about, how we're developing these techniques to do these in-vitro diagnostics.
Yes, and on the scale that we've talked about here.
And I think for all of you that come from Cornell, you got started in this business earlier than most other places the idea that microfluidics and nanotechnologies give you the idea of miniaturization and parallelization, the ability to integrate a multiplicity of chemical manipulations of the body make-up.
I can't just all-- [inaudible] half, obviously.
What we did, what I did, about three years ago was went around and visited a number of places that were doing nanotechnology on the West Coast, and the person who impressed me most of all those that I've visited was Jim Heath because in a second he got the idea that if we were to set up a partnership, the driving force in that partnership had to be the need for systems biology.
And Jim, that's actually really learned the systems biology very well, and he's been a marvelous partner.
In addition, we started with Steve Quake who is, really, as I'll say in a moment, finally have some things in microfluidics at Caltech, and-- or recently, has moved to Stanford where have continued collaborated with him.
And we set up with UCLA and Michael Phelps, some dual approaches to molecular imaging that I'll talk about a little bit later.
But the idea for in-vitro diagnostics that is to be able to do a thousand or 2,000 measurements on the fraction throughout the-- quoted through this microfluidics device.
And, you know, the questions that we have to worry about are, how can we make it scalable, how can we integrate all these functions together, how can we manufacture these things in a really large scale?
That is a problem.
Jim has just recently started to come at grips with that and-- and the protein-capture agents, antibodies aren't going to work.
So, what is going to work?
We'll talk about that.
Steve Quake really revolutionized the field microfluidics by introducing the idea that if you could have salt materials then that allows you to make constant values in mixing chambers which are the kind of the basic elements of doing the kind of, well, diagnostics that we weren't able to do in a completely integrated manner that runs many, many thousands of samples if necessary.
And the-- from Steve, we've actually adapted these microfluidics approaches at the institute, and Adrian Ozinsky recently has actually developed a theory [inaudible] microfluidics ELISA assay.
It has the ability to do totally remarkable things.
And here's the design.
We don't have to worry about it.
He can take a single cell, a macrophage, and he can measure from a single cell five different cytoplasms across five different time points.
So you can begin to do kinetic measurements of key molecules.
And by the end of another six to eight months help with the device, it will be able to make a 100 such measurements if we can get the capture agents.
And again, that's a point I'll return to.
But to give you an idea of the sensitivity of the analysis that we're talking about we're seeing with some analysis, it is after [inaudible].
And back to what Adrian has done recently is to lyse a single macrophage, dilute it a hundred fold, and show by real-time PCR, not even microfluidics techniques, that he was able to see 20 key genes that were present at the RNA level in that particular cell type.
So, I think we're going to be able to create, in a very short time, very powerful methods so that we have the information content of single cells and we'll be able to look at the molecules at very low levels.
What Ken Keith [phonetic] has done in the meantime is develop means for manufacturing in massively parallel process, really high quality nanotubes.
And, in fact, more recently, he's developed a method where he thinks he can really stamp these things out at very high-rate of reduction.
And of course, the real key for these nanotubes is to be able to functionalize each of them individually with the different kind of capture agent.
And to give you a scale, you can put about a thousand of these nanotubes in the diameter of a typical eukaryotic cell, 10 microns or so.
What Jim has pioneered is attachment chemistry.
So he can take protein-capture agents such as antibodies and fix them to these nanotubes.
And once you've done that, when the ligand binds to the antibody, it changes the capacities of the nanotube in a manner that's proportional to the amount of ligands so you can actually look at the concentration of proteins in this case that would be present in the blood.
And in a similar thing, he's develop, and in fact in human chemistry, the nucleic acids so you could measure for example, RNAs, based on those nanotubes.
But, you know, one of the really big limitations is where are we going to get 2,000 capture agents?
And what we've come across recently is a new type of chemistry called Click Chemistry that gives us a very powerful new approach to creating in a high tubular fashion our capture agents.
And this is a chemistry that was pioneered by Hartmuth Kolb who then was at the Scripps and now has moved to UCLA and he's working with us on these endeavors.
And the idea is you can use the protein molecule itself as a biological target to which you can affix two low-affinity binders in reducing peptides.
Right now, we're exploring aptamers for that.
And the idea is with the one low-affinity binder, you'll put a different series of extenders, and at the end of it, you'll put an [inaudible] compound.
And then at the second low-affinity reagent, you'll put a series of different extenders, and at the end of it, an [inaudible] compound.
And when you juxtapose with the right extensions these two reagents, the interaction which is highly specific, there was essentially no nonspecific interactions here occurs, you click these two things into place, and voila, what you've created is a lining reagent that is the multiple of the two affinities at each of the low-affinity binders.
So, it's really easy to find 10 to the minus 6 peptide binders.
It's really hard to find 10 to the minus 12.
But here, we can develop them very nicely.
And what Hartmuth Kolb has done recently is to take carbonic anhydrase and he's made a click high-affinity reagent using exactly the kinds of chemistries that I talked about before.
He's placed on it Fluorine 18 molecule and then we've used it in PET scanning in mice to show, with enormously high precision, that the high-affinity reagent localizes precisely where you would expect carbonic anhydrase to be.
And partly with this, actually created-- in the last-- that was done in less than three weeks to just to give you the scale-- time scale of things.
And he's, in the last six months, have created 10 such reagents, and he's never had a failure with the creation of these reagents.
So, we're actually in the process of setting up a copy that is going to quantitate on a very large scale this process.
And its first customer is going to be another company that's defining these molecular signatures we've talked about.
The proteins will need to be able to diagnose for-- looking at the disease process.
So, the idea then would be that you could generate these large arrays of molecules.
And in fact, the even greater vision is that you could not only create nanotubes that could interrogate the levels of a particular protein or messenger RNA, but you could create a nano-laboratory [inaudible] that will do five measurements that are really key to systems biology.
So, one is the idea that you can interrogate what you call behavior of individual cells using electrical measurement processes, hence you can do real time measurements.
And we've done this or adapt 10 different biological assays with individual macrophages.
So, it works-- it works just beautiful.
The second idea of course is that you can take the contents of the single cell and dump them on these functionalized nanowires and obtain the levels of protein and or messenger RNA that are present in those cell types.
And then of course, that you could do exactly the same-- dump the contents of the single cell on functionalized nano [inaudible] that my crew at Caltech is actually working on, and there we hope to be able to get a lot of specific protein-DNA interactions, transcription factor interactions, and of course, levels in protein-protein-protein-DNA interactions are a key measurements in generating these networks that we've talked about.
And the idea then is that we could use one of these integrated microfluidics devices to drive actually the process and operation of 500 of these little nano-laboratories.
So, you have the ability to have a very high [inaudible] production in the single cells.
And let me tell you, I think the ability to extract information from single cells is really going to transform biology in major ways because everything we've done today, with some minor exceptions, deals with populations.
And there are fundamental questions that can't not answered from population biology that I think are fabulous to all of you.
Now, I know you are working here at Cornell in devices that produce single molecule of DNA sequencing.
We're collaborating with a company that seem quite to set out to doing exactly the same kind of things.
And it's my own feeling that the future of DNA sequencing is going to be done by single molecules, and I think what we'll be able to set the model up so that they can be done in a massive and parallel fashion.
And quite obviously, if you're to do a billion single strands of DNA molecule from an individual for-- to fit to your own basis, you can have the entire genome and be able to do it quickly and inexpensively, and that's going to happen.
And enormous effect upon medicine and we'll talk about that in a few moments.
But I do want to stress really one point and that's this idea that I see coming over the next 10 plus years, this digitalization of biology and medicine, the ability to analyze the informational content of single cells, and or single molecules.
And my only feeling is this digitalization is going to have heart-breaker in fact on our future than the digitalization of information technologies because it, you know, deals with fundamental essence of what we are and how we're going to deal effectively with disease in the future.
And I would say, I think it is these approaches that are going to turn around the steep costs for-- of what it costs to get medicine to people in such a way that in time we're going to be able to export these to the third world, the developing world countries.
I thought a lot about these [inaudible].
Let me give you an example of studying an [inaudible] disease, this is prion disease, and its approach to drug targets and early diagnosis.
Now, as some of you may know, prion is really a fascinating disease.
We started studying it with Stan Prusiner in 1983 where you see plans for protein for the first time and [inaudible] forming of the gene, and Stan ended up getting the Nobel Prize in 1996 for this disease.
But it is a fascinating disease in many ways.
It's a disease in which a normal protein becomes folded in a different configuration.
And in doing so, we have some two fundamental new properties.
Number one, it has a property to go back and catalyze the transformation of normal prions to their disease form.
And number two, it has the ability to catalyze the technology that lead the means to have parts of the nervous system and so forth.
The problem with this-- in this whole process was to say the onset of prion disease from a systems point of view.
And initially, we did a really simple experiment, that is, we took a normal mice and we took a mice that had been infected with prion and compared the early and the late stages of these different diseases.
And we did it in a kinnetic sort of way, but the early studies were really interesting.
So, we looked at message level in the brain and spleen.
Those are the two organs where you have the largest concentration of [inaudible] of prions.
And we both-- in the blood got it-- got it.
So, protein is the change.
And what we asked was for the Venn diagram, we overlapped interval between the blood messages that changed and the plasma proteins that changed.
And likewise, the spleen messages and brain messages that changed.
Now, why are we interested in four sheer different in the blood and the brain and the [inaudible] between the spleen and the brain?
We are interested because that is a selected filter that asked us to post the following question.
Because we know that the prion gene is in the center of a protein and gene regulatory network, that is the heart of this pathologic disease process, how many of those proteins [inaudible] messages and the overlap integrals mapped into this key network containing about 130 genes, 25,000 per cellular levels?
And the answer was, essentially all were mapped into this network.
And the simple point is, if we use these blood molecular fingerprints with the appropriate biological filtering, it's going to point us to the primary networks where we can begin thinking about new strategies that we'll talk about later in generating drugs.
Now, what we've done more recently is look in exactly the same thing, how not but one strain but four different inbred strains of mice infected with prions that they have different incubation times and we've looked at many different timeframes.
And the only point that I want to make here is that 'cause we've looked at four different inbred strains, we can ascertain how many genes change in all of these for the patients, but even more important, we can ascertain how many messages changed are shared by all four strains.
That is, we can use this multi-strain comparison to deal with the genetic polymorphisms that are rampant in mice.
And of course, the implications through humans are quite obvious.
But anyway, we can work with this score of 1,100 or so changed proteins.
And what we can do kinetically is map across time to the expression patterns of each of these genes has in the context of a whole series of functionalities, growth functionalities that we generated.
And there is enormous amount of information about pathologic disease process in here, but-- and I'm not going to talk about it 'cause, again, that's a lot of discussion.
But it is a very powerful tool for understanding the entire process of disease and for being able to generate networks that will allow you to work across time at the nature of the difference of the change for particular functionalities.
The accumulation of the scrapie protein, the neurotoxicity and degeneration-- degeneration that they cause, and or some of already know, regular events that are deviated by these little processes.
So you can come to understand in really deep way the nature of the pathophysiology, and it never-- it's never ever before been accessible.
And of course the challenge in dealing human disease is where we can get this kind of genetic data.
And there are a few places, but it's obviously not easy to do.
Now, the final point that I would make is if we'd look at these 1,100 differential expressed genes, several hundred are actually expressed well before in the mouse as you can detect either histologically or clinically any scientific disease.
So, we'll be able to do early diagnostic serum.
This is really an important in mad cow disease for example, because in mad cow disease what happens is they now have to go so far into the pathologic process which that almost automatically count the end out, destroying all the cattle in the herds, but if you can do it very early before they have the chance for cross infection, you can take a very different strategy.
And of course, you have this whole Variant Creutzfeldt-Jakob disease, the human equivalent of the prion disease and so forth.
What I want to make-- a point now, is how we have focused in a such an integrative way on taking this systems approach of disease in the institute, and I can say 12 out of 12 faculty members are participating in a quality way.
The validation of these fingerprints and the correlation of the fingerprints with the disease and developing the various technologies, we need to read the fingerprints.
Actually were doing some really interesting [inaudible] imaging, I'll talked about that in a moment, developing new mathematical models for extracting the maximum information from blood fingerprints.
All the classic ways of doing multi-parameter analysis will only give you minimal amounts of information because the analysis has to be done in an entirely new context, that if we know the systems within which these perturbed are operating and we have to bring back information into the multidimensional analysis, and developing new computational networks for building methods for building dynamic networks, and systems approaches and model or some stuff, learning how to discover for our targets, and really how to reengineer proteins.
And those are-- those are all endeavors that are starting to get people right now.
But as a consequence born out of these new system, these newer systems, newer disease, and with this new kinds of measurement technologies and so forth.
I'm arguing that we're going to have this new kind of testing that will merge over the next, well, a few to 20 years or so that is predictive, preventive and personalized.
So, by predictive, we may have-- my prediction in 20 years everybody in this room will have the genome sequence determined, and we'll be able to use these new multi-variant systems analyses to correlate on your variant genes a future of probabilistic health history.
And of course [inaudible] me is to able to do that in a way that we can actually say how hard we've come down that path.
So we'll have, I think, in the five to eight years, everyone will have at home a little device that can prick your thumb and take a drop of blood and make 2,000 measurements and send that through wireless into a server and have it analyzed and send it back to you and your physician has an email that says you'll probably do it again six months or see your oncologist.
Now, just to give you my idea of how powerful it may be-- we'll be able to perch this whole endeavor.
If we can read these blood molecular fingerprints-- as I've said we can do early diagnosis, disease through application, all disease progression, all response to therapy, early detection and adverse reactions.
In fact, it's going to be terrific for titrating drug.
That was the something drug companies can't do at all now.
But I want to say one more thing to have the institute and start thinking about this, it is always really hard to do biology in humans because we can't manipulate them experimentally.
What these blood molecular fingerprints give us the ability to do is interrogate virtually the entire human at will in response to the normal stimuli that you go through.
That is, how do all these systems change over 70 years of aging?
How do these systems change if you prolong hormonal treatment for menopause?
I mean, so, we have a completely new approach to doing biology in human organisms.
So I think we just have to apply our imagination.
Once we have the disease of course, treat it with stem cell.
Prevention is all about using these systems approaches and being able to design for us that can do one of two things.
One has the ability to cure diseases you already have.
I've talked a little bit about how we can get to the systems and do we prevent it and thinking about doing that.
But, two, I think will, in the future, actually be able to design drugs that can prevent the permutation of the disease, the perturbed networks in major diseases.
So, my idea is in the next eight to ten years we'll have this nano-laboratory process to where we can do a needle biopsy on you.
We can take a thousand normal cells and analyze them and a thousand cancer cells and analyze them, and we can build the networks and see which are the perturbed networks.
I mean, if you permutation of those individual cells and articulate and delineate into more detail the nature of those networks, and that will give us enormous insights.
And key the mobile point which you'd like, which is perturbed, which is-- the network factor are more normal function.
And once we generate those drugs by quite obviously we have to be able to visualize, or it can be done into [inaudible].
We think for reasons I'll show you in just a moment that we're going to have very powerful approaches with PET scanning to do that.
So, in conjunction with GM and [inaudible], we've used microfluidic approaches to create a lab-- a discovery laboratory which can invent in an incredibly short periods of time new drugs for PET scanning.
Once you figured out the chemistry, then you can affix the microfluidics device and parallelize it.
And, actually, on the microfluidics device, enable the dosages that we'll need for reasonable numbers of human patients.
And, of course, the Quick Chemistry is going to be the absolute key to repeat for a diagnosis because it will let us interrogate any of the wide variety of different informational molecules that will be so important.
Now, here you have an interesting permutation network system I didn't discuss for lack of [inaudible] needs, but it is this graphical network I talked out.
But here, the red dot indicates this was a gene that we knocked out, and the grayscale, the white and the black, are part of the levels that [inaudible] there are present in the corresponding system.
But what's interesting, let's suppose that this was a protein that you-- or a drug that you wanted to map out the activity of this drug in the system.
But if you look at other [inaudible] around here, what do those represent?
Those represent cross reactivities of that drug.
So we have with these systems approaches the ability to deal upfront from ever getting the patients with the systems that might be susceptible to cross-reactivity in drugs and so forth.
So, I would just guard you that if you want to think about the process of drugs discovery, systems biology can do two things really well: identify protein targets and identify the side effects.
And that those two alone, I think can transform the whole drug discovery process and reinvent the same drugs.
So, predicting medicine-- preventive medicine can lead to a personalized medicine the fact that we're all uniquely, genetically separate from the same [inaudible], then it points to the fact that we're susceptible to different combinations of diseases, obviously mandates the idea that we're going to have to create it in an individual fashion.
And as I said last night, I think this is a kind of medicine which will emerge over the next five to 20 years.
It's really going to transform the entire healthcare industry.
So, a big pharma-- I mean, it isn't doing a very good job in making drugs.
They're constantly expensive.
Their inhibition, are they going to be able to adapt to these new approaches and so forth?
And the same is true of many aspects that the healthcare industry, the health insurance, HMOs, all of these other kinds of things like Academia, ethical schools are probably going to have to change how they teach physicians, maybe we're going to have to have two different branches of physicians, supposed that are fundamentally engaged in research and obvious their fundamentally engaged in practice and so forth.
So, just as Gordon Moore back in 1965 have made this very startling prediction that lead to the digitalization of information technology, namely the number of transistors should be put on computer chip would double every 18 months in advance.
So, we see in biology an exponential increase in all types of biological information.
And of course the fundamental question is how do we convert that information into knowledge about the organism?
And I think it's going to be dynamic, these system approaches with which I hope hypothesis and discovery driven, global, quantitative, integrative.
It'd be dynamic [inaudible] scale that that we have talked about, but they do have to exist in retrograde for us just on every kind of environment.
And a final point that I would make is just to reiterate this idea again that educational institutions, academic institutions really will want to throw in some ways systems biology to an equal-- a very powerful importance for biology that most biologist actually carry out because they are enormously synergistic in many ways and in fact we collaborate probably with 20 or 30 laboratories.
Now, if they're doing small biology and they've enriched what we can do by going in to a level of detail that couldn't be approached on the [inaudible] that we've enriched what they've done by giving them a more global approach to some fundamental concept questions that they want to ask.
So the collaborators that are listed here in all cases, they are almost all really cross-disciplinary groups that have gather to do these things.
And in all cases, they gave fundamental contributions to their individual stories each of which could have been an entire, you know, this lecture here.
So with that I'll close and answer your question.
[ Applause ] [ Noise ] Yes?
[ Inaudible Remark ] [Inaudible] what we don't know, and I understand that in some degrees, but you said that's not important, but others say predicting side effects-- for instance it only happens-- it's not just the drug might be off target, it might have an off target effect, but the on target effect might have, you know, effects that we aren't aware of as far as the model is [inaudible].
So do you that these kind of, you know, schematic network models up the connections really [inaudible] from the attribute based on whatever practical knowledge in terms of [inaudible]?
>> So, I think the question is that our knowledge of signal transduction is embryonic is very growing and that's certainly true.
And are the models that we have now for predicting side effects and so forth really might have been useful in medicine, and I think the answer is yes.
I think for being able to manipulate networks or for being able to look at side effects, we don't have to understand all the biology, we just have to have assays that say, well, you're really have to change these things out here.
And frankly I don't think you'll reengineer the side effects.
But I'll think you'll say "That blood is so [inaudible] we got to go at another one that has more circumvented kinds of side effects." The other thing I would say about signal transduction is I think the reason we haven't progressed very far is we haven't used these systems approaches for it.
So, Alan Aderem, my colleague at the institute, has looked at the macrophage in terms of activation of pole receptors with specific stimuli and so forth.
And there it's beautifully demonstrated the complex nature of the signal transduction networks that operate, but moreover he's beginning to get some insights into how specificity is obtained in these seemingly totally interconnected kinds of networks and so forth.
But I think just because of extensity of interconnection that we're going to understand the signal transduction networks in a deep way, you'll have to take a more global-- a more systems kinds of approaches for doing that kind of thing.
You know, I have to go at the airport soon, so, okay two more questions.
You know, I think I have to run.
Yes?
[ Inaudible Remark ] Right, so the question was how do you determine when a network is significantly changed, and that's a really good question.
So, what we're doing is in these, we're actually looking at this kaleidoscope of utilization network where we knock out each of the individual elemental members, okay?
And we're looking at the proteins that these secretes, from those [inaudible] and we're trying to see if we can read back then the nature of the changes that we encountered are correct.
So, we're trying to do that on the model system that take it into a more complicated system.
Yes?
[ Inaudible Remark ] [ Multiple Speakers ] [ Inaudible Remark ] >> Oh no. No, no.
I didn't go in to all of the subtleties.
No, I think compartmentalization, globalization of proteins, their modification as they move from one compartment, those are all really absolute critical things in understanding biology.
So, there was no intent except to make the slides on it shorter 'cause there are other things you could put in where you'd get to the higher levels, too.
So, [inaudible].
So, no I think-- I think-- [Inaudible Remark] Yeah.
>> How do you even get that kind of data [inaudible]?
>> Well, you know, you've already seen out there a number of ways to get it.
I mean, you can use to screen for us the protein, and you can look very beautifully at what-- I think those are [inaudible] with regard to expression is.
So, I think that's the beginning of how will it begin to think about globalization and so forth.
But I think there are other things that one can do with nano particles in globalization [inaudible] in very interesting ways too.
So, I think they're full of variety approaches that are [inaudible] very important for that.
Okay. If it's hospital-- [Inaudible Remark] >> Oh, there's one more, maybe one more question.
[ Inaudible Remark ] No, we can do it either way.
We can do it either way but it-- and either way has different kinds of damages, let's just leave it at that.
[Inaudible Remark] Okay?
Okay, well thank you very much.
[Applause] [Silence]
About the speaker
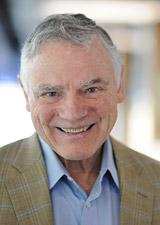
Leroy Hood
President, Institute for Systems Biology
Dr. Hood’s research has focused on the study of molecular immunology, biotechnology, and genomics. His professional career began at Caltech where he and his colleagues pioneered four instruments—the DNA gene sequencer and synthesizer, and the protein synthesizer and sequencer—which comprise the technological foundation for contemporary molecular biology. In particular, the DNA sequencer has revolutionized genomics by allowing the rapid automated sequencing of DNA, which played a crucial role in contributing to the successful mapping of the human genome during the 1990s. In 1992, Dr. Hood moved to the University of Washington as founder and Chairman of the cross-disciplinary Department of Molecular Biotechnology. In 2000, he co-founded the Institute for Systems Biology in Seattle, Washington to pioneer systems approaches to biology and medicine. Most recently, Dr. Hood's lifelong contributions to biotechnology have earned him the prestigious 2004 Association for Molecular Pathology (AMP) Award for Excellence in Molecular Diagnostics and the 2003 Lemelson–MIT Prize for Innovation and Invention. He was also awarded the 2002 Kyoto Prize in Advanced Technology and the 1987 Lasker Prize for his studies on the mechanism of immune diversity. He has published more than 600 peer-reviewed papers, received 14 patents, and has co-authored textbooks in biochemistry, immunology, molecular biology, and genetics, and is a member of the National Academy of Sciences, the American Philosophical Society, the American Association of Arts and Sciences, and the Institute of Medicine. Dr. Hood has also played a role in founding numerous biotechnology companies, including Amgen, Applied Biosystems, Systemix, Darwin and Rosetta.